
The main difference between a neural network and a deep learning one is the addition of multiple neural layers. Neural networks are inspired by the human brain, and so are deep learning networks. This situation makes neural networks especially interesting for IoT data processing. So why am I talking about neural networks at all? We live in an IoT world with tremendous amounts of data available and also (nearly) unlimited computational power available by using the cloud. The only catch with neural networks is the tremendous amount of computational resources and data that they need to perform well.
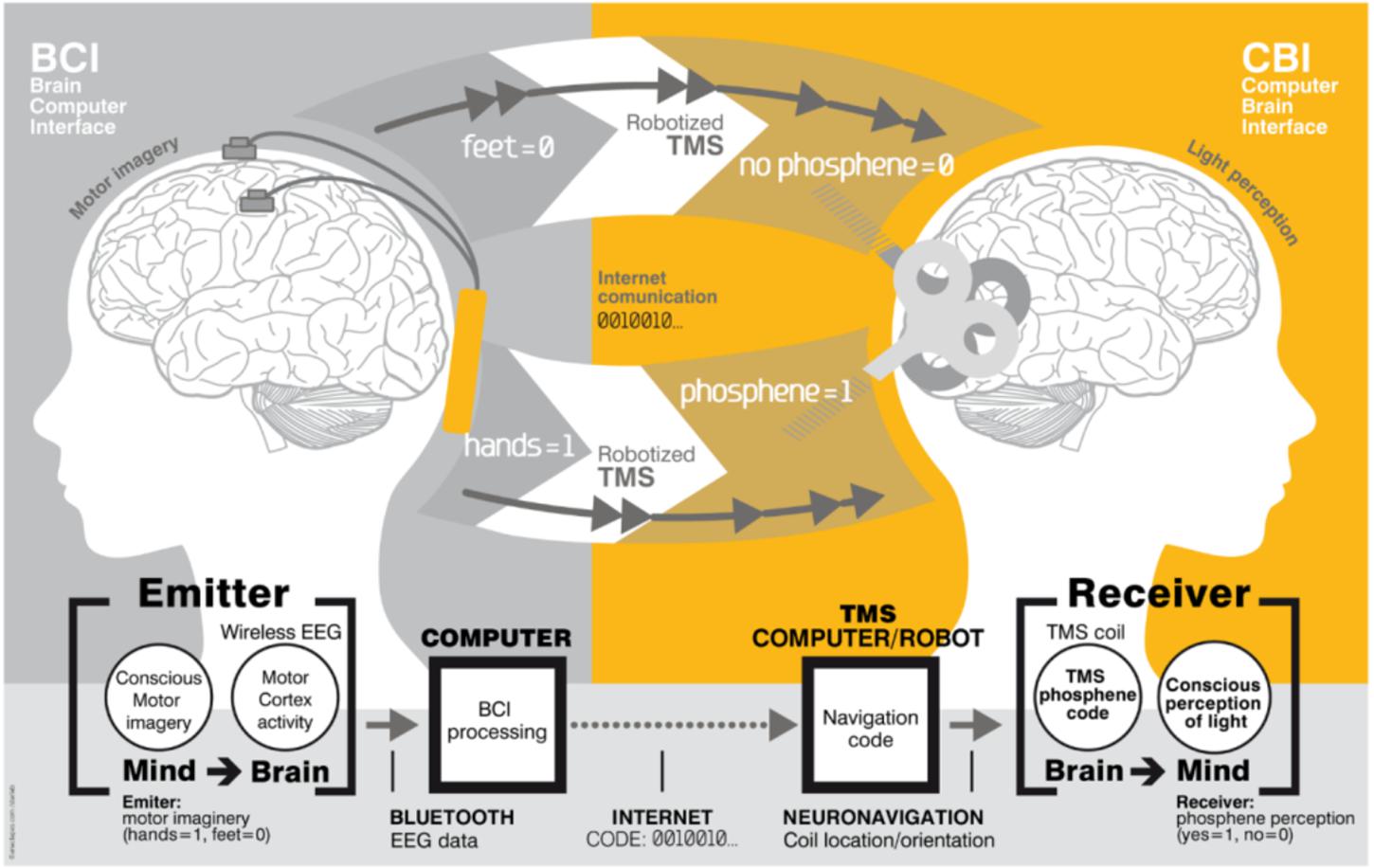
It is highly adaptable to data, and it is able to learn any hidden mathematical function between the data and the outcome. The component that such an algorithm produces is called a machine-learning model.Ī special type of machine-learning algorithm is a neural network. In this example, a (supervised) machine-learning algorithm is capable of considering all this data (and much more) to learn and predict faults from pure data.

The root cause for this situation might be the result of a broken bearing in a machine. As you might guess, a high temperature and high vibration led to a faulty part. In this highly artificial example, the numbers speak for themselves. It contains historic data on different parameters, measured observations on a manufacturing pipeline, and a binary outcome. It is a statistical model (black box) that in contrast to a physical model (white box) has been trained with data to learn a hidden pattern. If a cognitive system is based on models, you need to look first at what a machine-learning model is. From machine learning to neural networks and deep learning
#Ihbm 2018 human brain mapping how to#
In the coming weeks, I will present three different tutorials about anomaly detection on time-series data on Apache Spark using the Deeplearning4j, ApacheSystemML, and TensorFlow (TensorSpark) deep learning frameworks to help you fully understand how to develop cognitive IoT solutions for anomaly detection by using deep learning. In this article, I try to help you make that leap. To understand a cognitive system that uses IoT sensors and deep learning analysis, you first need to understand the leap from advanced machine learning to neural networks. In my last article I stated cognitive computing is not just human-computer interaction (HCI), it is advanced machine learning driven by powerful algorithms (models) and nearly unlimited data processing capabilities. Cognitive systems can learn and interact naturally with humans to gather insights from data and help you to make better decisions.

And guess what, all these devices are continuously generating data data that is useless unless you can analyze it.Ī cognitive system provides a set of technological capabilities such as artificial intelligence (AI), natural language processing, machine learning, and advanced machine learning to help with analyzing all that data.
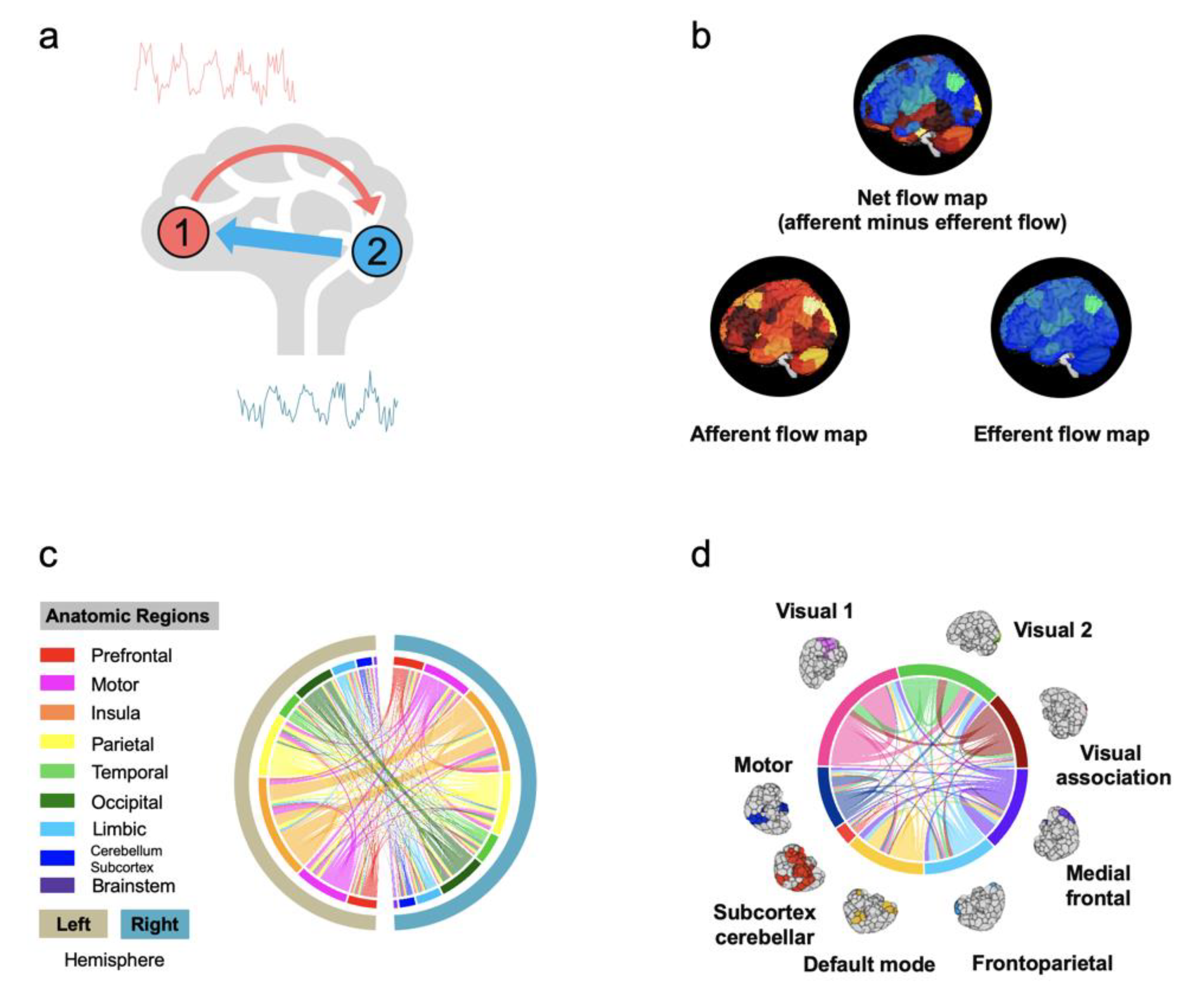
Even if over-estimated, soon the number of IoT devices will exceed the number of humans on the planet. A single amino acid change from isoleucine to valine at position 88 in VP3 attenuated neurovirulence by reducing virus replication in the brain and spinal cord of infected mice.Although predictions are always controversial, Gartner says that there are 8.4 billion connected IoT devices in 2017 (not counting smartphones) and some analysts say that by 2020 there will be 50 billion.
#Ihbm 2018 human brain mapping series#
A series of chimeric and point-mutated infectious clones were constructed to identify viral elements responsible for the distinct virulence. Sequence analysis revealed several conserved genetic changes among these virus strains: nucleotide positions 107 and 648 in the 50-untranslated region (UTR) amino acid position 88 in VP3 1, 148, 282 and 283 in VP1 22 in 2A 47 in 3A. Among the EV-D68 strains tested, strain US/MO-14-18949 caused no observable disease in this mouse model, whereas the other strains caused paralysis and death. We found that neonatal type I interferon receptor knockout mice are susceptible to EV-D68 infection via intraperitoneal inoculation and were able to recapitulate the paralysis process observed in human disease. This study aims to identify potential viral virulence determinants. Enterovirus (EV)-D68 has been associated with epidemics in the United Sates in 2014, 20.
